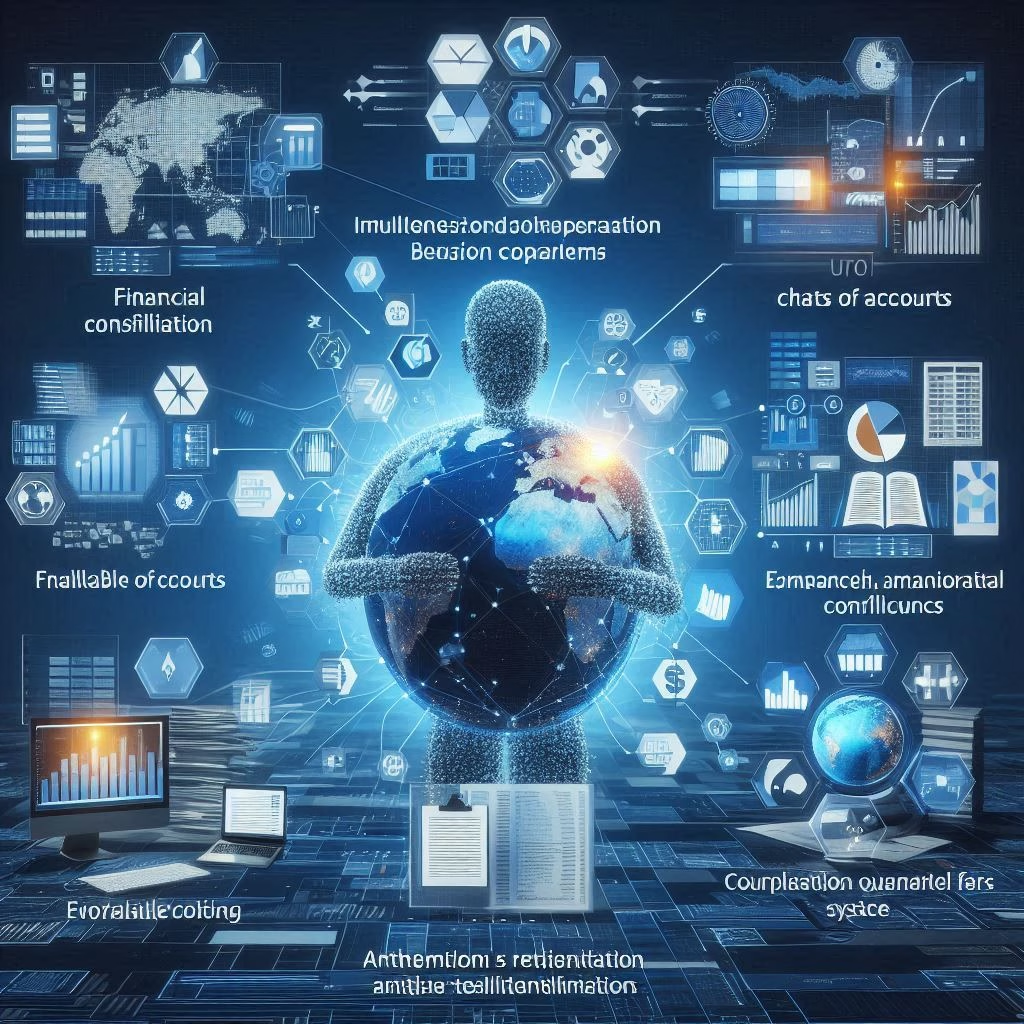
Introduction: A New Era in Finance
Financial consolidation has traditionally been a complex, manual process requiring significant coordination across departments, countries, and systems. As businesses grow through acquisitions or international expansion, consolidation becomes even more challenging. Multiple entities mean multiple sets of financials, charts of accounts, currencies, and compliance requirements. Artificial Intelligence (AI) and Machine Learning (ML) are now stepping into this space—not just to automate tasks but to enhance decision-making and uncover insights previously buried in spreadsheets.
This blog explores the role of AI and ML in financial consolidation—what they mean, how they’re already being used, what practical implementation looks like, and where they might take us in the future.
Understanding the Basics: What is AI and Machine Learning?
AI is a broad term used to describe machines or systems that can perform tasks that would normally require human intelligence. This includes recognising patterns, making decisions, processing language, and learning from data. Machine Learning is a subset of AI focused on algorithms that can “learn” from historical data to make predictions or decisions without being explicitly programmed.
In the context of finance, these technologies are not just buzzwords—they are tools that can automate processes, improve accuracy, and even offer new strategic insights. For example, an AI model might be trained to detect anomalies in journal entries, while a machine learning algorithm could forecast future revenue based on years of financial history.
Large Language Models (LLMs), like GPT, have also emerged as powerful tools capable of understanding and generating human-like text. This opens up possibilities for AI-powered assistants in finance departments that can answer questions about trends, variances, or even draft commentary for reports.
Where AI Can Help in Financial Consolidation
Mapping Charts of Accounts Automatically
One of the most tedious and error-prone tasks in financial consolidation is mapping subsidiary accounts into a common chart of accounts. AI can speed up this process by analysing naming conventions, historical mappings, and transactional patterns to suggest or even automate account mappings. For example, if a subsidiary uses “1002 – Cash Bank 2” and another uses “Cash Secondary,” AI can infer that both map to a consolidated account like “1020 – Cash & Equivalents.”
Intelligent Currency Conversion
Converting data from various currencies is another routine part of consolidation. AI models can flag inconsistencies in exchange rate applications or detect outliers in converted figures. Even more, a system could automatically apply the appropriate rate (spot, average, or historical) based on the account type and period, while highlighting anything that requires manual review.
While real-time exchange rates may not be suitable for standard reporting due to volatility, AI can learn patterns over time and help users choose the best rate source or timing for consistency and auditability.
Detecting and Suggesting Elimination Entries
AI can assist in intercompany elimination by recognising reciprocal transactions. For instance, if Company A records an intercompany sale to Company B, AI can match the corresponding purchase recorded by Company B—even if descriptions or timings differ slightly. Over time, the model can improve its confidence levels, flagging high-probability matches and reducing the time accountants spend identifying and eliminating these entries.
Forecasting and Scenario Planning
Perhaps the most exciting application of machine learning in consolidation is predictive analytics. By analysing trends across subsidiaries and combining them with external data (like macroeconomic indicators), ML models can forecast future consolidated performance. Users can simulate different scenarios—like changing a product pricing strategy or introducing a new cost-saving initiative—and see the forecasted impact on EBITDA or net profit at the group level.
For example, a growing SME could use historical expense patterns to predict upcoming cash shortfalls, while a global corporation might model the impact of currency shifts on profitability by region.
Natural Language Explanations and Anomaly Detection
One of the more recent applications is generating plain-English summaries of financial results. Instead of writing commentary manually for board reports, AI can identify the key drivers of performance (e.g., “Revenue increased by 8% due to strong Q4 sales in Europe”) and provide insights into unusual fluctuations.
In parallel, machine learning algorithms can detect anomalies in data—like an expense item that’s unusually high compared to past periods or peer companies—and alert finance teams before the close.
A Practical Approach to Using AI in Consolidation
The most effective way to adopt AI in financial consolidation is to start small and build gradually. For instance, begin by automating account mapping suggestions, or let an ML model flag potential intercompany matches. These steps deliver immediate time savings and increase confidence in the consolidated numbers.
Finance teams should also integrate AI tools into their existing workflows. For example, use AI to prepare a first draft of consolidation journals or to highlight areas needing attention in the final review. Over time, as confidence in the models increases, their use can expand into more complex areas like forecasting and strategic reporting.
It’s also important to consider data quality. AI and ML models are only as good as the data they are trained on. Consolidated reporting pulls from diverse sources, so ensuring consistent, clean, and complete data is essential before automation can deliver its full value.
A company using a system like BrizoSystem, which already provides structured access to multi-entity financial data, is in a strong position to begin layering on intelligent automation and AI features.
What Could the Future Look Like?
The future of AI in financial consolidation is promising. As AI models continue to improve, we may see systems that can:
- Auto-detect all intercompany relationships and generate elimination journals with near-perfect accuracy.
- Continuously monitor data across entities for inconsistencies or risks.
- Build financial forecasts in real-time, adjusting dynamically as actuals come in.
- Offer conversational interfaces for executives to ask questions like, “Why did our consolidated gross margin drop in Q3?” and receive context-rich, data-backed answers instantly.
One possibility is the emergence of a financial co-pilot—an AI agent that understands your group structure, policies, and data, and assists you daily in preparing, reviewing, and interpreting consolidated reports. Imagine being able to say, “Create a forecast based on last year’s seasonality, adjusted for this year’s currency trends,” and seeing a fully formatted dashboard appear with explanations and action items.
Final Thoughts: Collaboration, Not Replacement
While AI and ML are incredibly powerful, they are not replacements for finance professionals. Instead, they serve as tools to reduce manual workload, surface insights faster, and help teams focus on what really matters—analysis, interpretation, and strategic decision-making.
Financial consolidation will always require human judgment. But with AI’s help, the process can be faster, more reliable, and more insightful than ever before. Whether you’re consolidating three entities or thirty, the future of finance is not just about automation—it’s about augmentation, where smart systems and sharp human minds work together.ation.